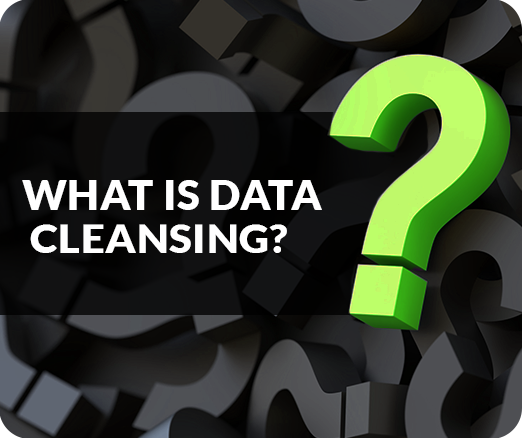
Data cleansing (also known as data cleaning or data scrubbing) is the process of identifying, correcting and/or removing duplicate, incomplete, corrupted, irrelevant, and incorrectly formatted data within a dataset so it’s consistent across different data sources.
The purpose of data cleansing is to improve the accuracy and reliability of data by removing errors and inconsistencies that can lead to incorrect analysis, insights, and decision-making. It is a critical step in data preparation for analysis, especially when dealing with large, complex, and disparate datasets such as retail point-of-sale data. Data cleansing can be performed manually but is best accomplished through automated tools and software that simplify and streamline the process such as VELOCITY®.
How is Data Cleansed?
The steps involved in cleaning data can vary depending on the nature and complexity of the data. However, some common steps involved in cleaning data include:
- Data Profiling: This step involves analyzing the data to identify any patterns or inconsistencies. It involves understanding the structure, content, and quality of the data.
- Handling Missing Data: In this step, missing data can be handled by inputting the missing values, removing the records with missing values, or using statistical techniques to fill in missing values.
- Removing Duplicates: Duplicate records can cause errors in data analysis and should be removed. Duplicates can be identified by comparing records on certain fields, such as name, address, or unique identifier.
- Standardizing Data: Inconsistent data can be standardized by transforming data into a consistent format. This can include converting dates to a standard format, correcting misspellings, or standardizing units of measure.
- Handling Outliers: Outliers are values that are significantly different from other values in the dataset. Outliers can be detected using statistical techniques and can be handled by removing the outlier records or by adjusting the data to account for outliers.
- Validating Data: Data validation involves verifying the accuracy and completeness of the data. This can include checking data against external sources or using business rules to validate data.
Why is Data Cleansing Important?
Poor-quality data can lead to inaccurate insights, unreliable decision-making, and wasted time and resources. By cleaning and standardizing data, organizations can improve the quality and reliability of their data, leading to better outcomes and more efficient operations.
How do you know if your data is clean?
There are several characteristics of high-quality data. Clean data is:
- Valid: It conforms to your company's or department's defined business rules or parameters.
- Accurate: The data reflects the information you require.
- Complete: The data is comprehensive with no gaps or missing information.
- Consistent: The data is consistent within the same dataset and/or across multiple datasets, i.e., it all matches.
- Uniform: All data conforms to the same unit of measure.
Want to learn how clean, harmonized retail sales and inventory data can make a difference in the growth and success of your company? Contact us today.