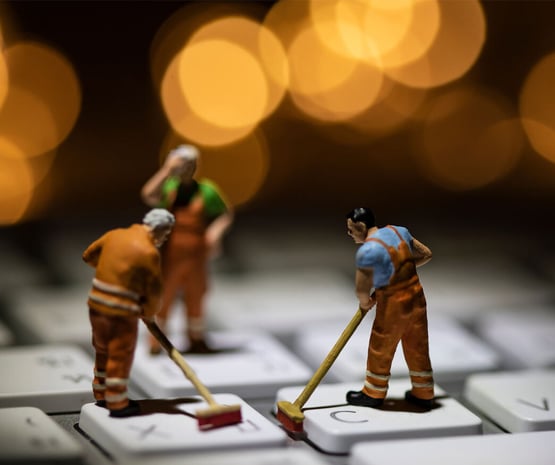
The Importance of Cleansed, Harmonized, Normalized Data
You know the old adage: Garbage in, garbage out. It even has its own abbreviation, “GIGO.” When you’re working with data—especially large volumes of point-of sale (POS), inventory, and enterprise data—what you put in absolutely determines what you’ll get out. If you don’t follow this critical rule in the right way, there can be serious negative implications, as bad data outputs could significantly skew your data reporting and analytics, derail business intelligence functions, or make your decisions flat-out wrong.
In the consumer goods and retail industries, data can be pretty dirty. Many times, manufacturers collect POS data from stores that either didn’t report sales, reported them incompletely, or reported them incorrectly. Although account teams can try to solve for these deficiencies by estimating the differences and buffering numbers, in the end they’re just hazarding a guess—and they’re wasting valuable time that could be better spent on leveraging data and not wrestling with it. This unreliable data levies serious consequences for the business decisions that follow, ranging from lost revenue and decreased profits to loss of market share and shelf space.
The importance of sound, precise data cannot be overemphasized.
How Can You Trust the Data?
Inaccuracy or incompleteness are the hallmarks of dirty data. Retail POS data software that tracks a sale incorrectly will generate inaccuracies in the data you receive. A technical issue that occurs in collecting downstream data might make the data incomplete. In both cases, the data may look correct when it isn’t. This is misleading—and can be catastrophic, not only to a manufacturer’s business but also to the retailers’ business and retailer relationships.
Fortunately, three important steps can ensure data is both accurate and complete: cleansing, harmonizing, and normalizing the data. When melding data from different sources, these processes integrate it in the most timely, effective, and useful ways.
What Does Data Cleansing Mean?
Often called data scrubbing (which is a subset of cleansing) or data cleaning, data cleansing resolves or removes duplicate, corrupted, inaccurate, missing, incorrectly formatted, out-of-date, or incorrect data to make the data more accurate and clearer. Data cleansing can be performed within a specific data set or while combining sets, but it’s especially crucial when combining sets since two or more sets create greater chances for redundancies and mislabeled products and information.
Specialized retail analytics software recognizes these discrepancies and automatically makes corrections by updating, changing, or deleting data. This “clean” data can then be used to inform important decisions, since it is now trustworthy, correct, and consistent.
View Infographic: The Importance of Data Cleansing and Harmonization.
What Does Data Cleansing Fix?
Data can be dirty for a number of reasons. Whether a cashier types an incorrect SKU at the time of the sale or two data sets have duplicate information, cleansing helps to fix a variety of issues:
Incorrect or Incomplete Data or Typos
During the data cleansing process, the software corrects typographical errors, inaccurate numerical entries, missing values, and syntax errors.
Irrelevant Data
It’s important to identify data that is not useful early in the process since out-of-date data or anomalies can affect analytic outcomes. The software recognizes this type of data and automatically removes it.
Redundant Data
Data sets often contain duplicate information; this is eliminated or integrated through filtering and deduping. If more than one record contains the same information, they can be combined into one.
Once cleansed, the data is complete, relevant, correct, and consistent.
What Does Data Harmonization Mean?
Another critical step in the process of preparing data for advanced analytics is data harmonization, which combines disparate data sources to ensure you’re working with “apples to apples” and all data make sense. A truly reliable software program will solve for differences in file types, data types, and data field and column naming conventions.
View Infographic: The Importance of Data Cleansing and Harmonization.
What Does Data Harmonizing Fix?
Integrating data from disparate sources, the harmonization process brings the following together in uniformity:
File Types
Many different file types can be combined. For instance, information from an Excel spreadsheet can be combined with information from a Google Sheets spreadsheet.
Data Types
Varying data sources, such as POS, EDI, syndicated, social ad, demographic, weather, and more, all have different ways of displaying information that must be formatted properly so that they can be combined correctly.
Data Field and Column Naming Conventions
Classification, also known as the field names of rows and columns, can vary greatly. If a promotion is labeled as field name “ItemA_Date” on a spreadsheet for your retail team and as field name “Marketing_Launch_Product_Name_Date” for your account team, harmonization will recognize these as the same classification and pull the information together under the same naming convention. This can be done with other fields as well.
Once harmonized, the data is uniform.
What Does Data Normalization Mean?
The process of data normalization ensures all data looks the same across all records and fields. This involves removing any duplicates or unstructured data (with no defined format or organization) and making everything uniform. Normalization ensures that URLs, names, street addresses, phone numbers, codes, and more correlate for quick computation. This is an important part of the process because many times these mismatches crop up when adding, changing, or eliminating information later. Normalization catches them early.
What Does Data Normalization Fix?
This process will work differently for different “mash-ups” of information; however, normalization does the work of data standardization—it keeps the same cases, adds dashes where needed, and expands abbreviations. For example:
- Ms. CHRISTINE becomes Ms. Christine
- 8008675309 becomes 800-867-5309
- SVP sales becomes Senior Vice President of Sales
Once normalized, the data follows the same style guidelines.
How Does Cleansed, Harmonized, Normalized Data Benefit Your Business?
IBM pins poor data quality to a whopping $3.1 trillion in losses per year for American businesses—due to factors such as decreased productivity, system outages, and increased maintenance costs. There is much at stake when so many strategic decisions and processes depend on having good data inputs and outputs.
With data cleansing, harmonizing, and normalizing, the data is manageable and trustworthy. Consumer goods suppliers and their retailer partners can easily use it to fuel insightful analytics that are accurate and informative. Businesses can consistently benefit from:
Stronger Sales and Marketing Strategies
With accurate and complete demand data, account and marketing teams can identify sales patterns and execute demand planning, promotional campaigns, and new product launches with confidence and success.
More Strategic Segmentation
Correctly organized and unified information provides teams the ability to perform aggregations by industry, title, or.
More Efficient and Effective Operations
A clear view of inventory and supply chain processes means that teams can proactively address on-shelf availability problems, delivery issues, and other headaches that increase costs, decrease revenue, and adversely affect customer loyalty.
Decreased Data Spend
Addressing data acquisition and management issues at the source saves the time and money it would take to remedy them in the long run.
Smarter Decisions, Period
With a bedrock of precise and reliable data, account teams and other departments can build the scaffolding of smarter business strategies.
Time Savings
These data management processes allow large amounts of data to be synthesized swiftly and with accuracy. Once data is prepared correctly, IT and account teams won’t need to organize or translate it again.
Expanded Space
Duplicate data is surprisingly digital space-heavy, stealing precious real estate in databases and draining processing power. Systems run faster and more efficiently when unnecessary data is removed.
One Version of the Truth
By combining various data sets and integrating them as “apples to apples,” an enterprise gains one version of the truth that all teams can rely on.
Greater Enterprise-Wide Adoption of Data
Clean, reliable data is powerful when it’s put to work. As more of the organization sees its potential, they’ll be encouraged to trust and adopt it, which will benefit the entire organization.
Good Governance
Trustworthy data throughout an organization underpins effective data governance for a more streamlined organization that’s also in compliance with data privacy and protection laws.
View Infographic: The Importance of Data Cleansing and Harmonization.
Seeing is Achieving
It’s easy to see how cleansed, harmonized, normalized data is essential to providing the foundation for smart business decisions throughout the enterprise. When your data is in the same language, it can be effectively leveraged for excellent reporting, analysis, and business intelligence.
Good in, good out. It’s a MUCH better kind of GIGO.
To learn how automatically cleansed, harmonized, and normalized data can transform your business, get in touch with us today.