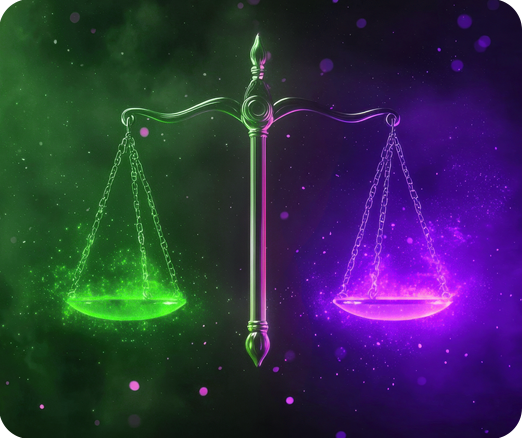
Retail Data Management: Key Factors in the Build vs. Buy Decision
In today’s digital economy, data has become the cornerstone of business success, particularly for Consumer Packaged Goods (CPG) companies.
Driven by e-commerce, omnichannel retailing, and an increasingly data-savvy consumer base, CPG companies and consumer brands are facing unprecedented challenges in collecting, managing, analyzing, and leveraging retail data.
As we move further into the 21st century, CPGs are increasingly confronted with the critical decision of whether to build or buy their data warehouses or data repositories. Unlike purchasing commodities such as cars or refrigerators, determining whether to build an in-house data repository or acquire a third-party solution is a complex and valid question. This choice has far-reaching implications, from cost efficiency and scalability to innovation and competitive agility.
This post will examine the strategic, financial, and operational factors that CPG executives need to weigh when choosing between building a custom retail data management solution in-house or purchasing a well-established third-party solution.
THE DEVIL IS IN THE DATA DETAILS
In 2024, the choice has evolved from the traditional "build or buy" dichotomy to one focused on "build and configure" versus "build from scratch." At a high level, the core SaaS components of any modern data solution remain the same—data import, processing, and export. Many organizations, when considering these at a superficial level, mistakenly believe they can easily build their own system. However, making an informed and successful decision requires attention to the finer details.
"In today’s environment, where the demand for robust and accurate decision-making has escalated, companies must move beyond traditional methods like EDI and transition toward APIs ..."
For example, importing flat files has long been a straightforward process, whether the format is EDI or a comma-delimited file from a retailer. This method has been common practice since the 1980s and remains relatively simple for anyone with a technical background. However, in today’s environment, where the demand for robust and accurate decision-making has escalated, companies must move beyond traditional methods like EDI and transition toward APIs and advanced data structures and file formats like Parquet.
A common misstep for those new to building their own repository is to focus on importing ALL available data without a clear plan for its utilization. The real challenge, though, is not in the ingestion of data but in selecting and processing the most valuable raw data, and then successfully harmonizing it for optimal usage.
Importing only retailer point-of-sale (POS) data should be the minimum requirement. Additionally, organizations should collect inventory data from retailers, particularly at the distribution center (DC) and store levels. The deeper insights derived from such data are invaluable to an entire CPG company, particularly for sales, marketing, supply chain, and finance teams.
The true complexity arises when importing and harmonizing additional, critical datasets, such as those related to trade promotions, demand planning, new product launches, weather, census, and category data. However, processing and harmonizing this information is worthwhile since it allows organizations to reduce costs through optimized processes.
IMPORTANCE OF AUTOMATING DATA HARMONIZATION
Data standardization is often confused with data harmonization, but the two are fundamentally different. While standardization is simpler and focuses on making data uniform, harmonization is more difficult because it involves integrating data from multiple sources into a unified, comparable view that enables better decision-making. Understanding the difference between the two—and how each can impact your data quality, insights, and decision-making—is important when deciding whether to build or buy your data repository.
Data harmonization involves both simple and complex tasks, such as standardizing raw data imports and conducting more intricate processes like cleansing and mapping. While many IT departments can handle basic tasks like importing and standardizing data, they often struggle with more sophisticated steps like identifying and addressing data gaps. Generic tools may assist with basic ingestion and mapping, but for more complex tasks, automation is crucial.
Automating these processes should be an organization’s goal, as complete automation reduces the risks (e.g., wasted time and money) associated with manual intervention and enhances efficiency, enabling an organization to focus on more strategic endeavors. If a company halts its automated processes after simpler tasks (i.e., data importing and standardization), its ability to generate meaningful insights and make impactful decisions will be compromised. Even worse, employing personnel who are manually trying to cleanse, map, and rectify data issues will prove to be cost-prohibitive even if using low-cost offshore resources.
WEEKLY OR DAILY DATA? STORE- OR CHAIN-LEVEL? THE CHOICE IS EASY.
When it comes to importing retailer POS data (the minimum data import requirement), operating at a weekly cadence or chain-wide level is outdated. Industry leaders are now ingesting daily SKU-level data across all SKUs and stores, both physical and online. This daily granularity allows for more flexible and accurate aggregation—whether by week, market, or chain—and leads to superior decision-making. Companies that still rely on monthly or weekly data processes risk falling behind competitors that have embraced daily data workflows as a best practice to evolve on their own terms.
"Leading brands ingest and integrate trade promotion, demand planning, weather, census, category,
and ERP-specific data into their decision-making processes."
Employing a data repository capable of handling daily SKU- and store-level data is no longer optional for brands aiming to remain competitive. Anything less than 100% coverage of SKUs provides only directional insights, which are insufficient for effective decision-making. Moreover, automating POS and inventory data ingestion and harmonization processes to manage 100% of the data at an item-store level ensures accuracy and minimizes additional time or costs.
The next phase in data ingestion should involve incorporating non-retailer data that has immediate strategic value across an organization. Leading brands ingest and integrate trade promotion, demand planning, weather, census, category, and ERP-specific data into their decision-making processes. Although importing such data is complex and presents challenges in mapping and comparison, the return on investment (ROI) is substantial. For instance, using daily item- and store-level data in trade promotion, demand planning, sales planning, or new product launches significantly enhances accuracy and optimizes results (e.g., sales growth, increased on-shelf availability, and inventory reduction).
Many organizations continue to operate and make critical business decisions based on weekly data, even though daily data has proven far more effective for strategic planning and optimization over the last 10 years. The shift toward daily data processes allows brands to transition from traditional push models—where products are moved into DCs and stores based on forecasts—to consumer-driven models where product pricing, product assortments, and retail replenishment are aligned with actual consumer demand.
Online sales operate with minute-level data, and in-store POS data is also collected in real time. Despite this, many companies still base their sales, marketing, supply chain, and investment decisions and processes on outdated weekly data and cycles. Forward-thinking organizations are already leveraging daily data and reaping the benefits of superior decision-making capabilities, outperforming their competitors, especially in better promotional ROI and optimized in-store inventory levels.
While ingesting and harmonizing daily data is undoubtedly more challenging than working with weekly data, the benefits are clear. The technical obstacles—data processing, storage, and speed—are well known, but the real challenge (and risk) lies in correctly mapping and comparing data. For instance, failing to accurately compare a product launch from a specific day this year to an aggregated weekly dataset from a previous year can lead to erroneous conclusions, suboptimal decisions, and lost sales, revenue, and loyal shoppers.
FROM EDI TO RETAIL DATA PORTALS: ARE YOU PREPARED?
Ingesting retailer data is merely the initial step. Retailers frequently modify non-EDI formats, necessitating continual updates to data extraction and ingestion protocols. For instance, some major U.S.-based retailers (in-store and online) have made many major changes to data specifications over the past 12 to 16 months. This has forced CPG data teams to adapt and adjust their data ingestion processes to ensure uninterrupted data flow, which can require many in-house IT hours and effort.
As the industry shifts from EDI to retail data portals and API-based data, these changes are expected to occur 3 to 5 times per year; however, CPGs must determine if they have the right resources to efficiently and effectively manage these portals. Companies that rely solely on EDI data may initially find it economical, but many now prefer retail data portals and API integrations because of the richer data available. Despite EDI’s continued presence, it remains outdated, with standards largely untouched for nearly two decades.
OPTIMIZED DATA SYNCHRONIZATION WITH RETAILER SYSTEMS
Precise synchronization between your SKUs and retailers’ items is crucial for effective data harmonization, as is aligning fiscal calendars to match the financial cycles of your retail partners. Companies often overlook this alignment and its importance—and the effort required to make it happen, leading to intensive reconciliation efforts later to ensure "apples-to-apples" comparisons.
Using a "lowest common denominator" approach during data harmonization and normalization yields the best analyses, comparisons, actionable insights, and results. Today, this level of granularity is generally daily/SKU/store, yet many systems offer only category/weekly/chain-level data. Attempting to substitute this data granularity sacrifices precision, particularly when trying to leverage retailer data for essential functions such as trade promotions, demand planning, new product launches, and optimization initiatives.
PUTTING DATA IN ITS PROPER PLACE
Once data is fully cleansed, harmonized, and normalized, the next step is to integrate it within your analytics ecosystem. Traditionally, suitable “landing spots” or repositories for this enriched data include:
- Data warehouse (either proprietary or vendor-managed)
- Data lake or comparable solution
- Visualization tools (such as Microsoft Power BI)
- Standardized reports, dashboards, or spreadsheets
However, clean, unified data can play a more vital role in advanced analytics tools and systems:
- Artificial intelligence (AI) and machine learning (ML) tools
- ERP platforms
- Stand-alone systems for warehouse management, trade promotion, and demand planning
data MAPPING AND EXPORT: NOT FOR THE FAINT OF HEART
Although exporting data to a spreadsheet might seem sufficient, it is generally inadequate for sophisticated decision-making. Similar to the concept that "importing is not integration," the same is true for exporting—it doesn’t ensure seamless integration.
"Building an in-house solution for data ingestion, cleansing, harmonization, normalization,
and exporting is a complex task for internal technology resources and not for the faint of heart."
For successful integration and data exporting, mapping field-level data accurately is essential to minimize risk and enable frictionless automation. Accurate mapping facilitates data harmonization and reporting, highlighting its importance in the data pipeline.
Building an in-house solution for data ingestion, cleansing, harmonization, normalization, and exporting is a complex task for internal technology resources and not for the faint of heart. Even well-established manufacturers have attempted this approach only to then limit the project scope or abandon it altogether due to resource demands. This knowledge of these failures provides insight into best practices, forming a preliminary checklist for data integration.
Some vendors may encourage a DIY approach, one that is centered around “buy the parts yourself and connect them yourself,” yet this often fails midway or incurs substantial costs. Outsourcing to lower-cost providers who claim to be “experts” can delay objectives and increase expenses, as they are not always cheaper or quicker in delivering services, which affects timely insight generation and decision-making.
OTHER INTEGRATION CONSIDERATIONS
Organizations must also address costs related to data mapping errors, infrastructure maintenance, expanding data fields, and functionality to meet evolving business requirements, as well as the common pitfall of collecting all available data indiscriminately without a specific purpose. While arguments for inexpensive processing power and storage are common, the strain of daily SKU-store-level data on those areas and the inability to correct poor data can slow decision-making, potentially incurring significant financial consequences. Using a reliable, configurable, SaaS-based solution can mitigate many of these hidden costs by handling infrastructure, data security, and scalability.
In summary, accurate data synchronization, comprehensive mapping, and careful selection of integration platforms are essential for enabling timely, data-driven decisions in a competitive market.
HARNESSING THE POWER OF PROVEN DATA PLATFORMS LIKE VELOCITY®
With proven, effective off-the-shelf solutions available, such as Retail Velocity’s VELOCITY® retail data platform, which meets the critical need for reliable retailer data, there is a strong case for using these existing options to generate actionable insights and support optimal decision-making. Therefore, a compelling rationale would be required to justify the internal development of a custom solution.
Even the largest consumer brands still struggle to cleanse and harmonize their data, a challenge that intensifies when trying to analyze data across multiple retailers. Therefore, implementing a robust, ready-made solution within several weeks or months offers the most compelling path forward.
A cloud-based data platform like VELOCITY offers unparalleled speed to market, cost efficiency, and scalability, allowing CPG companies and brands of all sizes to remain agile and competitive in an increasingly data-driven market. Moreover, the advanced analytics, security, and compliance features that come with VELOCITY provide a significant advantage over building an in-house solution or infrastructure from scratch.
Using pre-existing, configurable data adaptors for both online and in-store data can streamline data ingestion and significantly enhance decision-making speed. Moreover, an advanced, highly automated, and proven data harmonization and normalization engine is essential, as is the capability to map and remap data with precision. As AI integration increasingly shapes business landscapes, compatibility with AI tools becomes vital. Partnering with a solution provider like Retail Velocity that not only meets these criteria but also brings decades of expertise is likely the optimal choice for your company and its retail data, analytics, and insights needs.
With 30 years of CPG-retail industry experience and providing proven automated solutions and services that help consumer brands streamline their retail data collection and management efforts, Retail Velocity is well-equipped to be your strategic partner that can ensure you leverage the most accurate and reliable data and insights to grow your business profitably. Contact us today to learn more.